【Researchers from the Intelligent Manufacturing and Precision Machining Laboratory, have proposed a new algorithm structure called FIP (Fast Inertial Poser) based on six inertial sensors considering body parameters, which brings a new approach for deployment on mobile terminals.】
Motion capture is widely used in various fields such as virtual reality, biomechanical analysis, medical rehabilitation, and film production. Inertial sensor-based methods have significant advantages in capturing human motion in large-scale, complex multi-person environments due to their low environmental requirements and absence of occlusion issues. Sparse Inertial Measurement Unit-based methods have greater research value due to their simplicity and flexibility. However, such methods often rely on deep neural networks, which impose a significant computational burden on the devices. Improving the computational efficiency and reducing latency of these methods, and deploying them on portable terminals pose significant challenges.
Researchers from the Intelligent Manufacturing and Precision Machining Laboratory, Department of Mechanical Engineering, Tsinghua University, have proposed a new algorithm structure called FIP (Fast Inertial Poser) based on six inertial sensors, which brings a new approach for deployment on portable terminals. The method consists of two stages: joint position estimation and kinematic inverse solution. Compared to previous methods, the main reasons for the efficiency improvement of this method are: firstly, eliminating additional optimization designs; secondly, based on the "minimum measurement principle," enhancing the expression ability of the neural network structure by considering human shape parameters (height, leg length, single arm length, and sex), using kinematic inverse solvers and a shared model of different sensors; thirdly, removing the bidirectional propagation mechanism of RNNs. In the position estimation stage, FIP uses three independent recurrent neural networks (RNNs) to estimate the positions of leaf nodes and the rest of the nodes. To make the model inference process closer to the real physical process, FIP uses a sensor-shared integration RNN to estimate the positions of leaf nodes. In addition, for each RNN, human parameter information is input into the algorithm network through embedding.
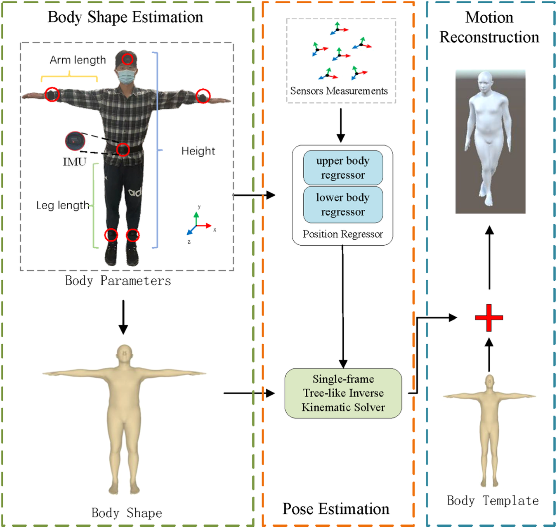
Figure 1. Illustration of FIP
The specific network design is illustrated in Figure 2, where the main sub-modules used are the unidirectional recurrent neural network (RNN) and the multi-layer perceptron (MLP). By inputting the human skeleton information into different modules to form human shape-related constraints, a special inverse kinematics solver based on the human kinematic tree is designed to help the model solve the rotation of joints in the current frame.
In experiments based on open-source datasets, FIP demonstrates reduced motion reconstruction latency and computation time while ensuring reconstruction accuracy. The computational time on a PC is only 2.7ms per frame, and it can run stably with a latency of 15ms and a frame rate of 65 FPS on the Nvidia NX2TX embedded computer. This provides a novel approach for real-time human motion reconstruction on mobile terminals.
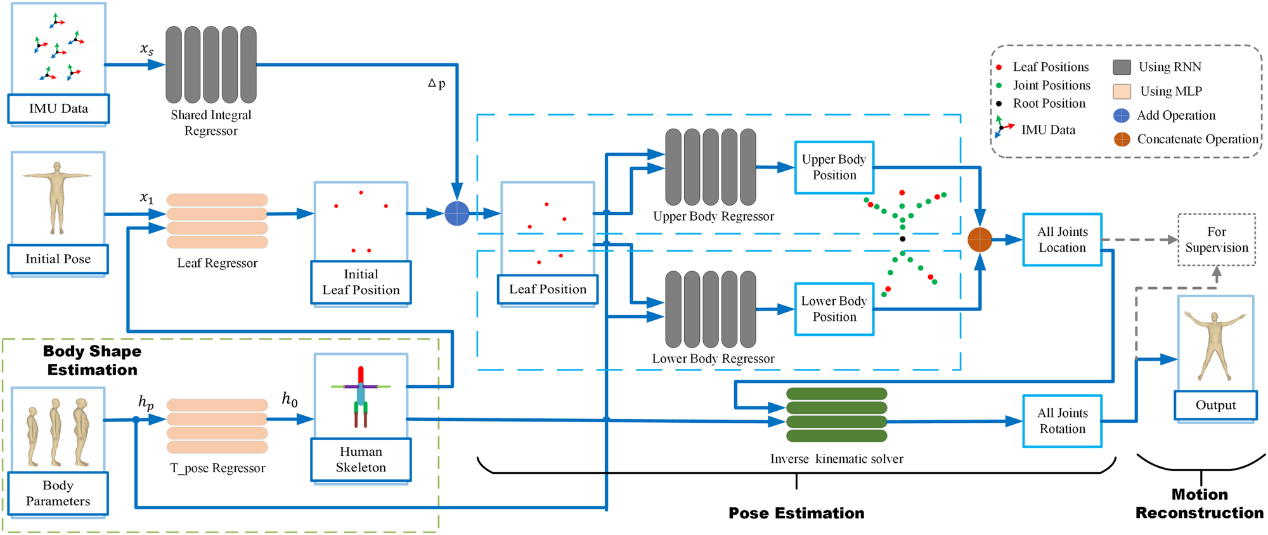
Figure 2. Pipeline of FIP
This work was published in the journal "Nature Communications" on March 18, 2024 with the title "Fast Human Motion Reconstruction from Sparse Inertial Measurement Units Considering the Human Shape". The first author is Xiao Xuan, a master's graduate from the Department of Mechanical Engineering at Tsinghua University. The corresponding author is professor Zhang Jianfu, from the Department of Mechanical Engineering. Gong Ao, an undergraduate graduate from the Department of Mechanical Engineering, along with Professor Feng Pingfa, Associate Professor Wang Jianjian, and Assistant Researcher Zhang Xiangyu, all from the Department of Mechanical Engineering, participated in the research work. The research was funded by the Guoqiang Research Institute, Tsinghua University.
Research link:
https://doi.org/10.1038/s41467-024-46662-5